The Singapore students using Cloud for smarter recycling
Coming up with big ideas in technology used to take the kind of time and money that only large companies had. Now open source tools—like TensorFlow, which provides access to Google’s machine learning technology—mean anyone with a smart concept has the opportunity to make it a reality. Just ask Arjun Taneja and Vayun Mathur, two friends and high school students from Singapore with a big ambition to improve recycling rates.
Arjun and Vayun realized that separating waste is sometimes confusing and cumbersome—something that can derail people's good intentions to recycle. Using TensorFlow, they built a “Smart Bin” that can identify types of trash and sort them automatically. The Smart Bin uses a camera to take a picture of the object inserted in the tray, then analyzes the picture with a Convolutional Neural Network, a type of machine learning algorithm designed to recognize visual objects.
To train the algorithm, Arjun and Vayun took around 500 pictures of trash like glass bottles, plastic bottles, metal cans and paper. It’s a process that would normally be laborious and expensive. But by using Google’s Colab platform for sharing resources and advice, the students could access a high powered graphics processor (GPU) in the cloud for free. They were also able to access Tensor Processing Units, Google’s machine learning processors which power services like Translate, Photos, Search, Assistant and Gmail. These tools helped their system analyze large amounts of data at once, so the students could correct the model if it didn't recognize an object. As a result, the model learned to classify the objects even more quickly. Once the Smart Bin was trained, all they had to do was place an object in the tray, and the system could predict whether it was metal, plastic, glass or paper—with the answer popping up on a screen.
Building on their successful trials at home, Arjun and Vayun showcased the Smart Bin with a stall at last week’s Singapore Maker Faire, and they continue to work on other projects. It’s a great example of how tools available in the cloud are cutting out processes and costs that might have held back this kind of invention in the past.
Demo :
Convolutional Neural Network (CNN)
This tutorial demonstrates training a simple Convolutional Neural Network (CNN) to classify CIFAR images. Because this tutorial uses the Keras Sequential API, creating and training our model will take just a few lines of code.
Import TensorFlow
Download and prepare the CIFAR10 dataset
The CIFAR10 dataset contains 60,000 color images in 10 classes, with 6,000 images in each class. The dataset is divided into 50,000 training images and 10,000 testing images. The classes are mutually exclusive and there is no overlap between them.
Verify the data
To verify that the dataset looks correct, let's plot the first 25 images from the training set and display the class name below each image.
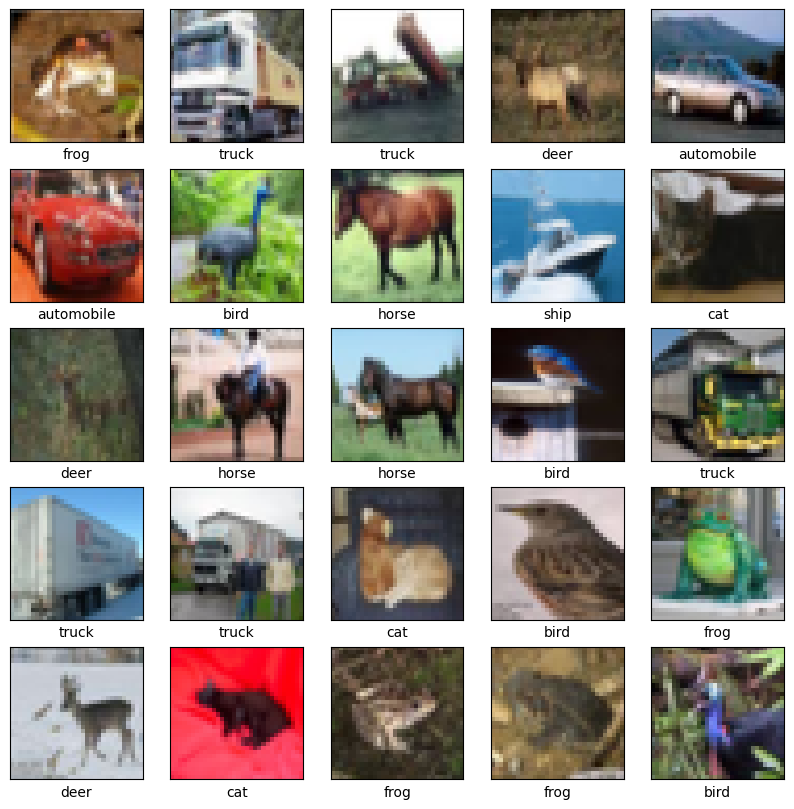
Reference : https://www.tensorflow.org/tutorials/images/cnn
0 Comments